- Published on
Scene Graph Parsing by Attention Graph
- Authors
- Name
- Martin Andrews
- @mdda123
This paper was accepted to the ViGIL workshop at NIPS-2018 in Montréal, Canada.
Abstract
Scene graph representations, which form a graph of visual object nodes together with their attributes and relations, have proved useful across a variety of vision and language applications. Recent work in the area has used Natural Language Processing dependency tree methods to automatically build scene graphs.
In this work, we present an 'Attention Graph' mechanism that can be trained end-to-end, and produces a scene graph structure that can be lifted directly from the top layer of a standard Transformer model.
The scene graphs generated by our model achieve an F-score similarity of 52.21% to ground-truth graphs on the evaluation set using the SPICE metric, surpassing the best previous approaches by 2.5%.
Poster Version
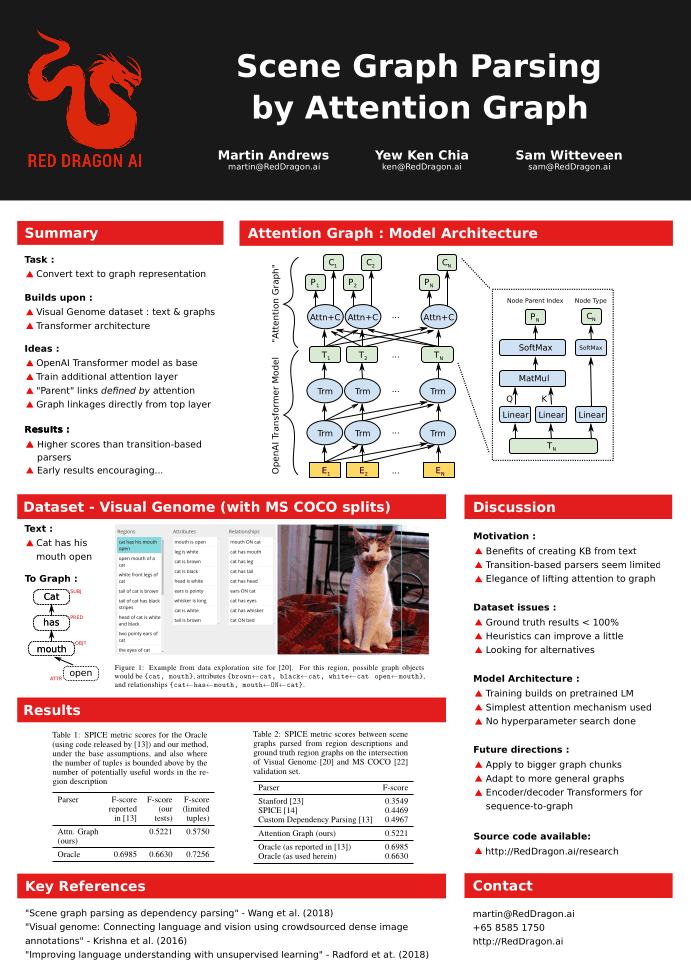
Link to Paper
And the BiBTeX
entry for the arXiv version:
@article{DBLP:journals/corr/abs-1909-06273,
author = {Martin Andrews and
Yew Ken Chia and
Sam Witteveen},
title = {Scene Graph Parsing by Attention Graph},
journal = {CoRR},
volume = {abs/1909.06273},
year = {2019},
url = {http://arxiv.org/abs/1909.06273},
eprinttype = {arXiv},
eprint = {1909.06273},
timestamp = {Wed, 18 Sep 2019 10:38:36 +0200},
biburl = {https://dblp.org/rec/journals/corr/abs-1909-06273.bib},
bibsource = {dblp computer science bibliography, https://dblp.org}
}