- Published on
Multi-Hop Inference Explanation Regeneration by Matching Expert Ratings
- Authors
- Name
- Martin Andrews
- @mdda123
This paper for the workshop Shared Task was accepted to the Textgraphs-15 workshop at COLING-2020 in Mexico City, Mexico.
Abstract
Creating explanations for answers to science questions is a challenging task that requires multi-hop inference over a large set of fact sentences. This year, to refocus the Textgraphs Shared Task on the problem of gathering relevant statements (rather than solely finding a single 'correct path'), the WorldTree dataset was augmented with expert ratings of 'relevance' of statements to each overall explanation. Our system, which achieved second place on the Shared Task leaderboard, combines initial statement retrieval; language models trained to predict the relevance scores; and ensembling of a number of the resulting rankings.
Poster Version
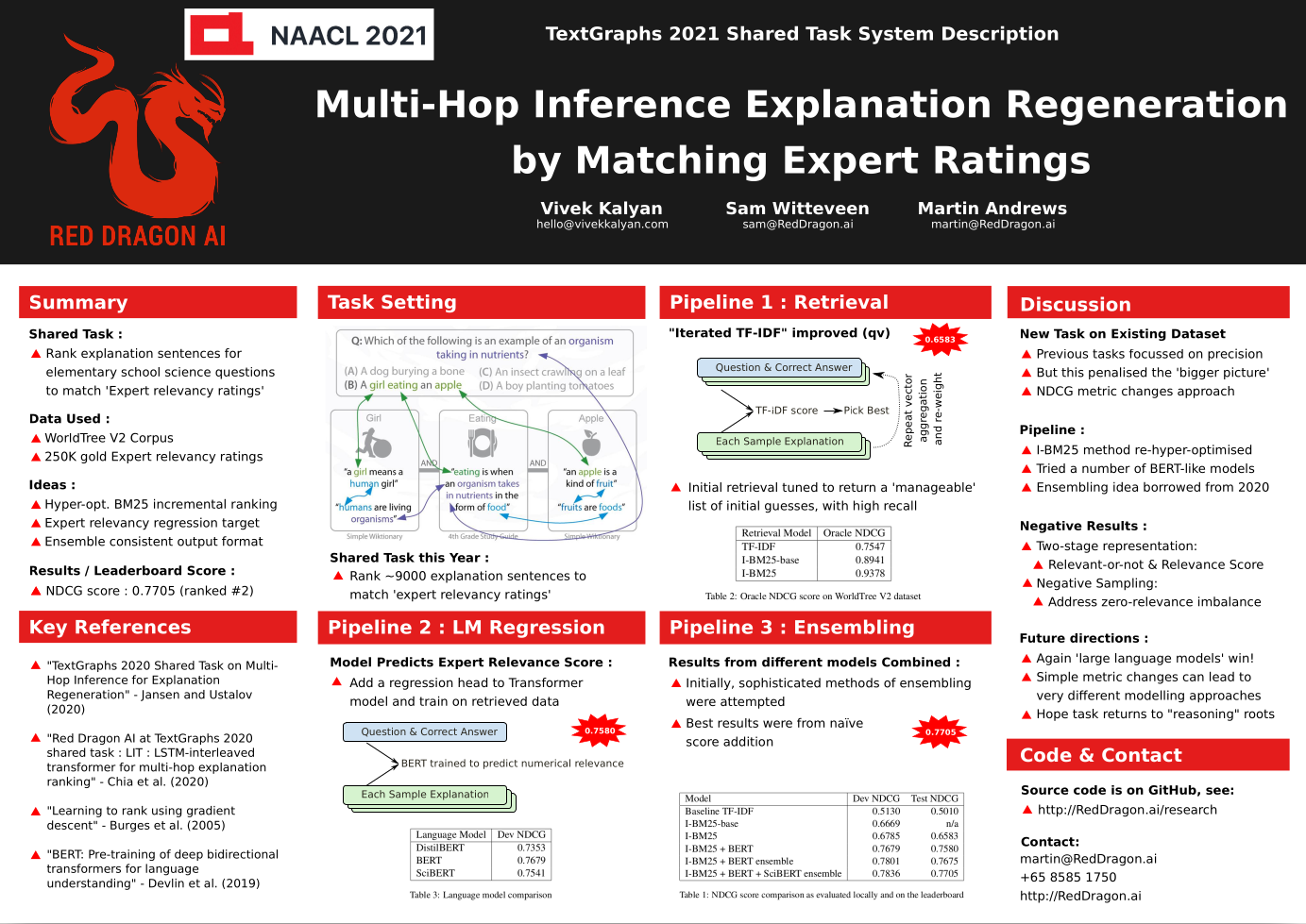
Link to Paper
And the BiBTeX
entry for the arXiv version:
@article{DBLP:journals/corr/abs-2107-13031,
author = {Vivek Kalyan and
Sam Witteveen and
Martin Andrews},
title = {Red Dragon {AI} at TextGraphs 2021 Shared Task: Multi-Hop Inference
Explanation Regeneration by Matching Expert Ratings},
journal = {CoRR},
volume = {abs/2107.13031},
year = {2021},
url = {https://arxiv.org/abs/2107.13031},
eprinttype = {arXiv},
eprint = {2107.13031},
timestamp = {Fri, 30 Jul 2021 13:03:06 +0200},
biburl = {https://dblp.org/rec/journals/corr/abs-2107-13031.bib},
bibsource = {dblp computer science bibliography, https://dblp.org}
}