- Published on
TensorFlow @ PyData SG
- Authors
- Name
- Martin Andrews
- @mdda123
Google just released a fantastic-looking deep learning library called TensorFlow
, complete with tutorials, and model-zoo-like examples.
Fortunately, the framework is very reminiscent of Theano
, and has a Python front-end over a computation graph construction machine in C++ / CUDA (no OpenCL as far as I can tell).
Presentation Link
Because it's new, and Python-related, I gave a "Lightning Talk" at the November meeting of the Singapore PyData group.
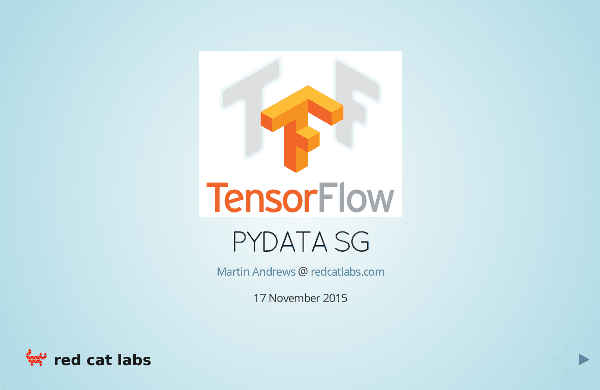
If there are any questions about the presentation please ask below, or contact me using the details given on the slides themselves.
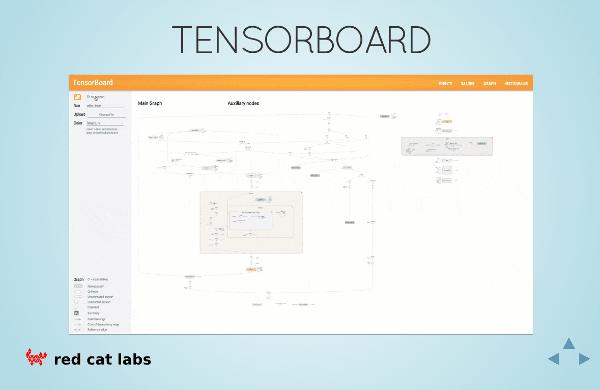
#Slide Notes (outline)
These are the notes I put together for myself before preparing the slides. Almost everything made it into the presentation, but only because I split the positives into 2 slides, and the not-so-positives into 4 slides...
Positives
Cross-platform : CPUs, GPUs, Android, iOS (soon), etc
Open Source (Apache 2)
- and Google actively reviews and responds to PRs
Theano-like definition and optimisation of calculation graph
- backend in C++
Python is first-class citizen (other is C++)
TensorBoard (images?)
Hiring tool
"Lowlights"
There are several points worth highlighting:
Nvidia devices supported much have 'Compute Capability' >= 3.5
- This includes 900-series cards, and Titan, and the 'K cards'
- But not 700-series (apart from 750), nor Amazon EC GPUs
- "A g2.2xlarge is a downclocked GK104 (797 MHz), that would make it 1/4 the speed of the recently released TitanX and 2.7x slower than a GTX 980."
Not many ops available in GPU
- Vector embedding example can't be run on GPU, for instance
- Theano can do this
- Vector embedding example can't be run on GPU, for instance
No OpenCL
Memory hungry - far prefers a 16Gb machine to an 8Gb one
Inefficient operations
- No in-place ReLU, for instance
C++ build environment requires
bazel
which is a Java-based horror storyPR submission process is via (painful)
Gerrit
rather than GitHubLegacy Nvidia drivers :
- 7.0 (rather than 7.5) for the main driver
- 6.5 (rather than 7.0+) for
cuDNN
, which is now 'archive', so not even supported
No distributed computation (yet), even though that's what Google uses
Only Python and C++ APIs (others will be 'community implemented')
Incorporates something like
fuel
(fromblocks
) as a data-feed engineWhole approach requires implementing many 'client' operations on the TensorFlow 'server' side
Currently targets Python 2.7 (though 3.3+ looks like it's coming soon)
Would definitely benefit from 'deep learning library' like
lasagne
theras
author has already stated that they'll target TensorFlow