- Published on
LIT : LSTM-Interleaved Transformer for Multi-Hop Explanation Ranking
- Authors
- Name
- Martin Andrews
- @mdda123
This paper for the workshop Shared Task was accepted to the Textgraphs-14 workshop at COLING-2020 in Barcelona, Spain (held online).
Abstract
Explainable question answering for science questions is a challenging task that requires multi-hop inference over a large set of fact sentences. To counter the limitations of methods that view each query-document pair in isolation, we propose the LSTM-Interleaved Transformer which incorporates cross-document interactions for improved multi-hop ranking. The LIT architecture can leverage prior ranking positions in the re-ranking setting. Our model is competitive on the current leaderboard for the TextGraphs 2020 shared task, achieving a test-set MAP of 0.5607, and would have gained third place had we submitted before the competition deadline.
Poster Version
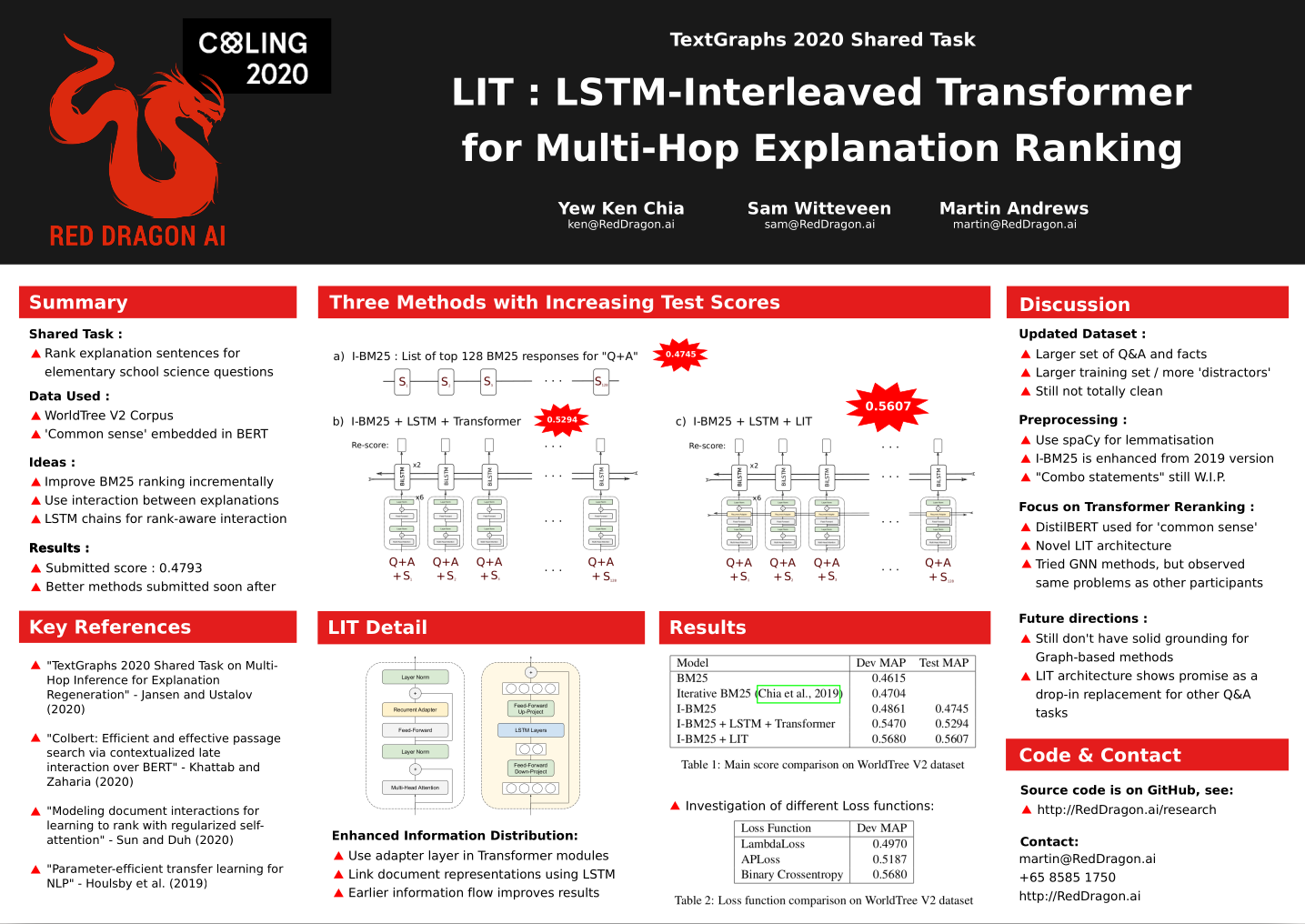
Link to Paper
And the BiBTeX
entry for the arXiv version:
@article{DBLP:journals/corr/abs-2012-14164,
author = {Yew Ken Chia and
Sam Witteveen and
Martin Andrews},
title = {Red Dragon {AI} at TextGraphs 2020 Shared Task: {LIT} : LSTM-Interleaved
Transformer for Multi-Hop Explanation Ranking},
journal = {CoRR},
volume = {abs/2012.14164},
year = {2020},
url = {https://arxiv.org/abs/2012.14164},
eprinttype = {arXiv},
eprint = {2012.14164},
timestamp = {Tue, 05 Jan 2021 16:02:31 +0100},
biburl = {https://dblp.org/rec/journals/corr/abs-2012-14164.bib},
bibsource = {dblp computer science bibliography, https://dblp.org}
}